Producing data science techniques & machine learning models
Shelter helps millions of people every year struggling with bad housing or homelessness through their advice, support, and legal services. They exist to defend the right to a safe home. Because home is everything.
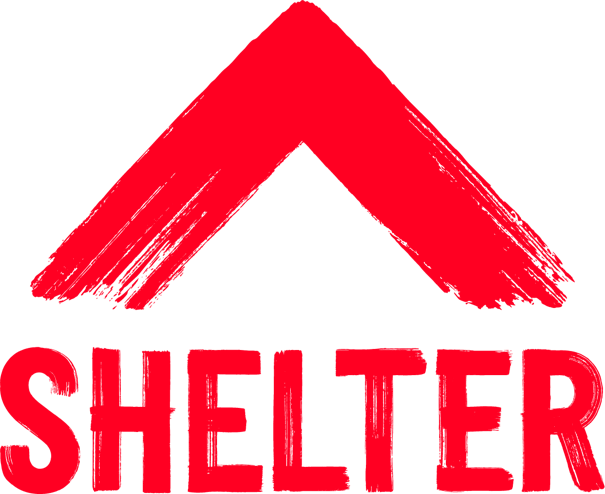
The Problem

Shelter has a number of channels through which users can contact them, with one such channel being a live chat feature on the website.
The Client Data Insight team at Shelter wanted to better understand how their service users were utilising their services by analysing transcript data from live chat sessions. The team would then use these findings to see how Shelter could better understand the needs of their users and assess what improvements could be made to their services.
How We Helped
The Solution
The Curve worked with Shelter to understand the goals and constraints for the project. From early conversations, The Curve identified a desire to use Python-based tooling, as this fits closely with the skills of the team at Shelter. Shelter is also working towards a more cloud-based strategy for their data.
As a result, the goal was to produce data science techniques and machine learning models that could be used independently by the Client Data team at Shelter, but which could also be deployed into Azure and utilise the various benefits of Azure ML.
The team at Shelter wanted to build a range of data science capabilities that would allow them to:
- Identify Key Phrases, Locations and Organisations
- Determine the issues and housing context from conversation transcripts
- Identify if a service user had been “put at ease” during the course of a conversation
The Curve took Shelter through natural language processing techniques that could be used to provide statistical insights into key phrases and organisation names of significance that appeared in the transcript data. Throughout the project, The Curve and Shelter worked together on a collaborative basis utilising The Curve’s technical and data analysis expertise, and the subject matter expertise of the Client Data Insights team at Shelter. After a number of iterations, a statistical-based approach was used to extract key phrases, nouns, locations and organisation names.
The team at Shelter also had a more ambitious goal of trying to determine the issues and housing context of a web chat conversation. They were able to identify metadata in web chat transcripts which we were able to use as a data labelling source to train a machine learning model or prior transcripts that would be capable of identifying the: Goal, Problem Area and Tenure.
Working together, we were able to develop models that on average were able to provide an accurate assessment of Goal, Problem Area and Tenure ~85% of the time. This is a strong result given the unstructured and free-form nature of web chat conversations.
Their Thoughts
Dean Robinson -Client Data Insight Manager, Shelter
“This project has helped to massively accelerate the team’s capabilities in terms of the tools that we have been able to apply in various new ways and has helped us to support the web chat and other teams within Shelter to get more value from their data and a richer understanding of the help they provide to their clients”
This work was made possible by the generous support of the National Emergencies Trust, who funded Shelter England as part of a wider partnership with Shelter Scotland, Shelter Cymru and Housing Rights Northern Ireland to support people facing homelessness and bad housing during the Coronavirus pandemic. We remain incredibly grateful for their support.
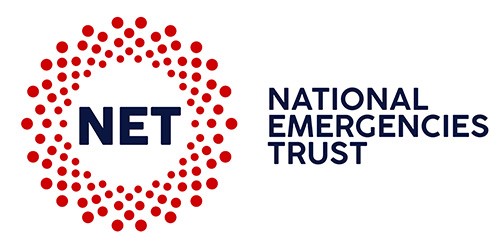